본문
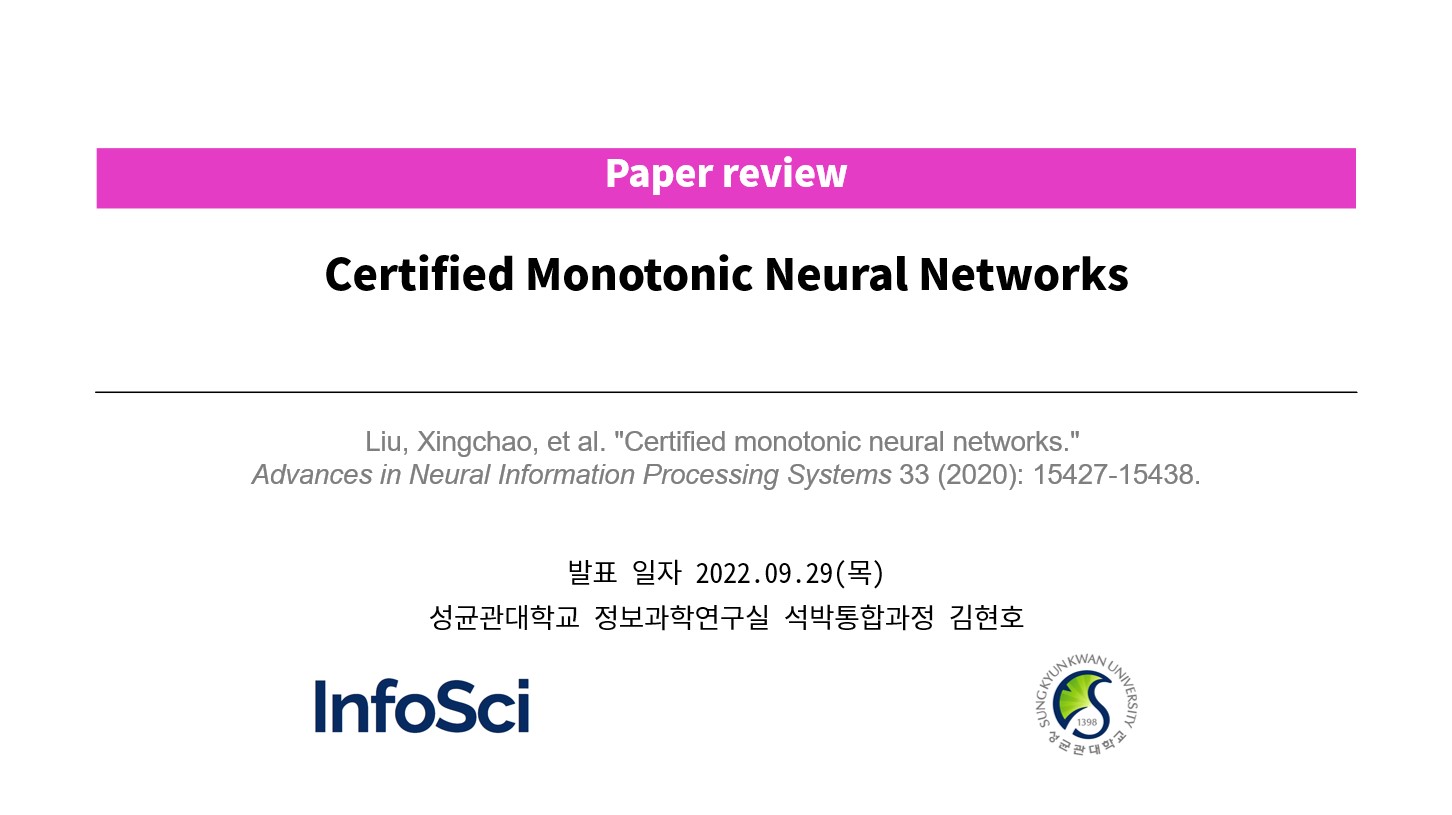
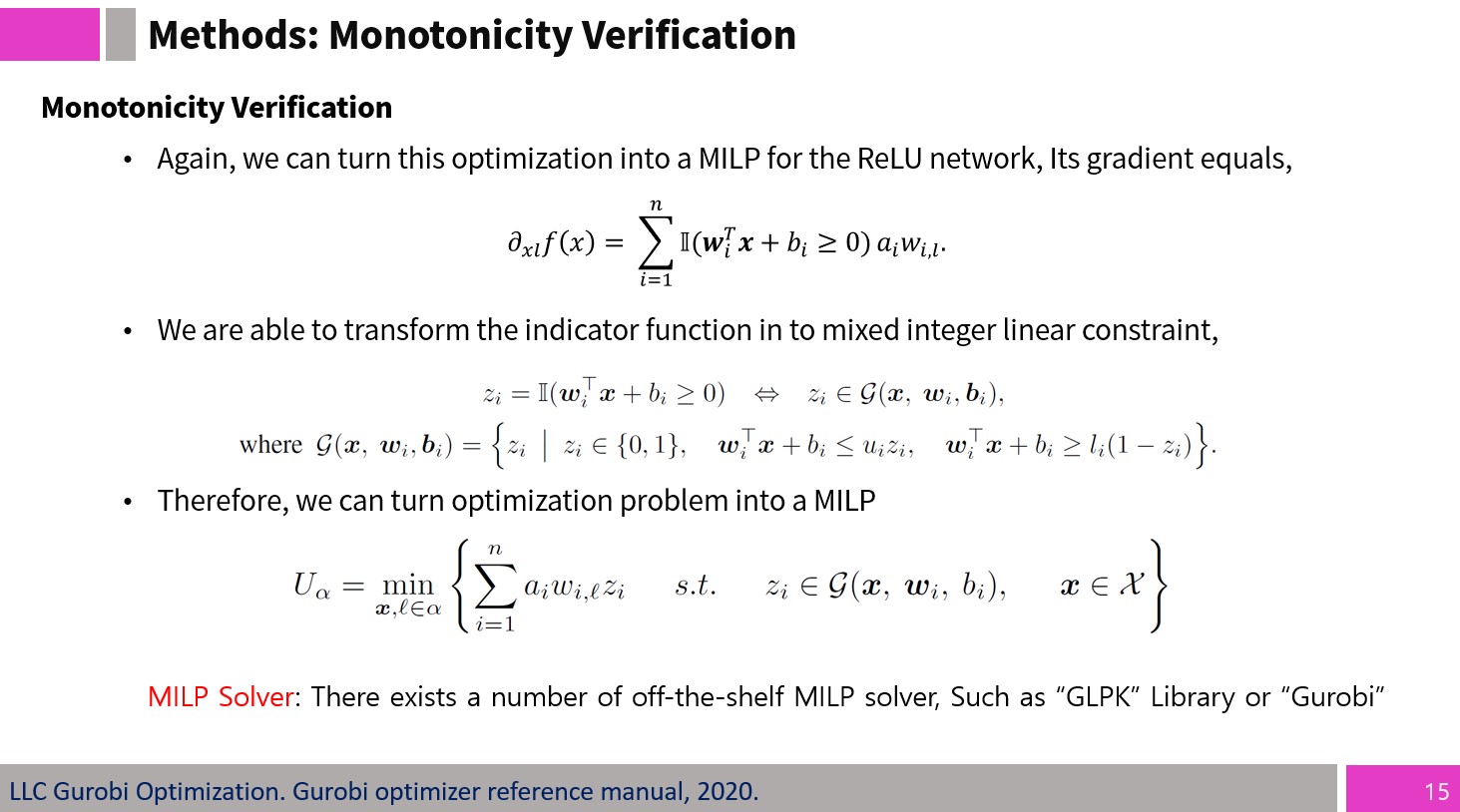
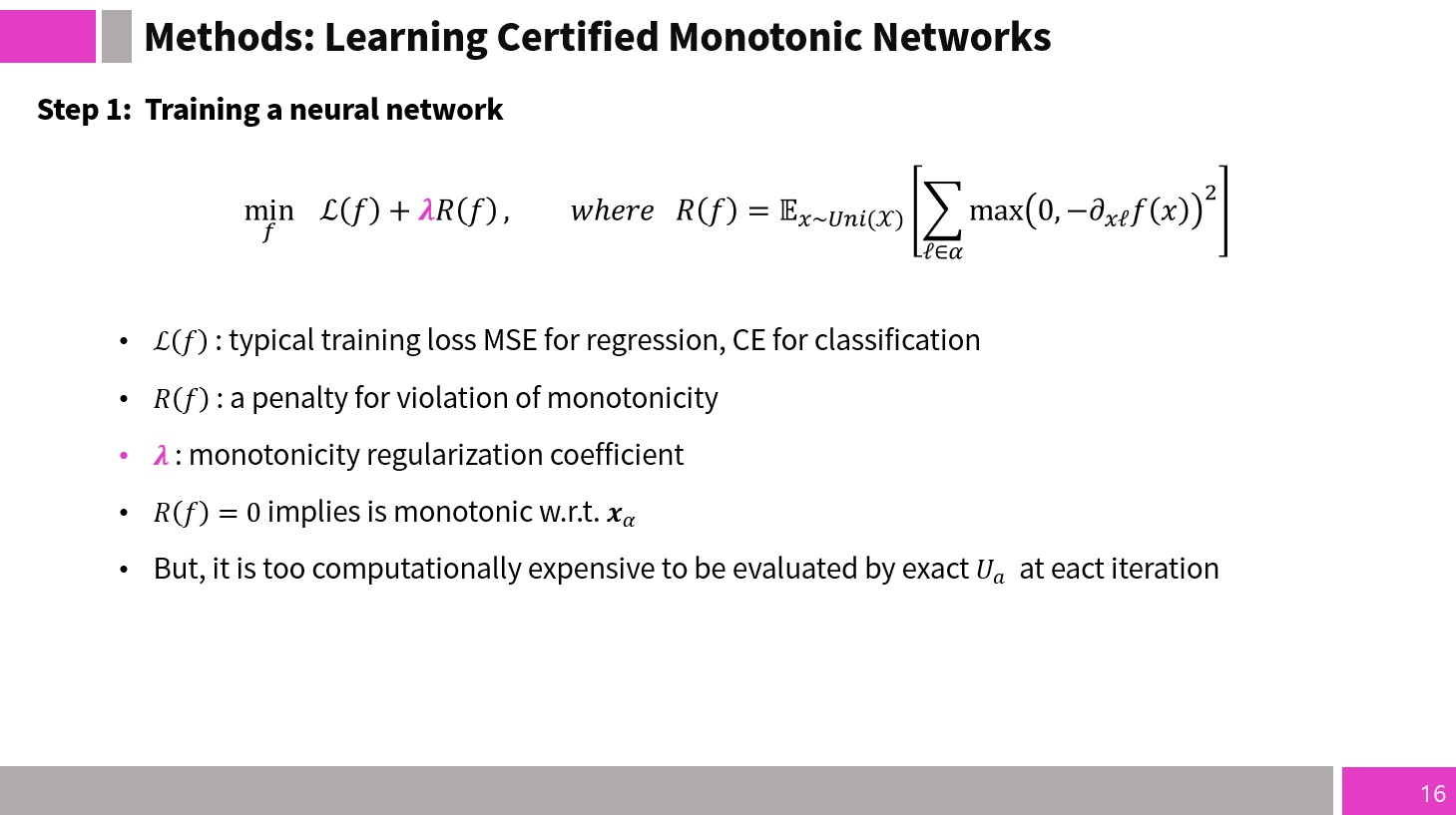
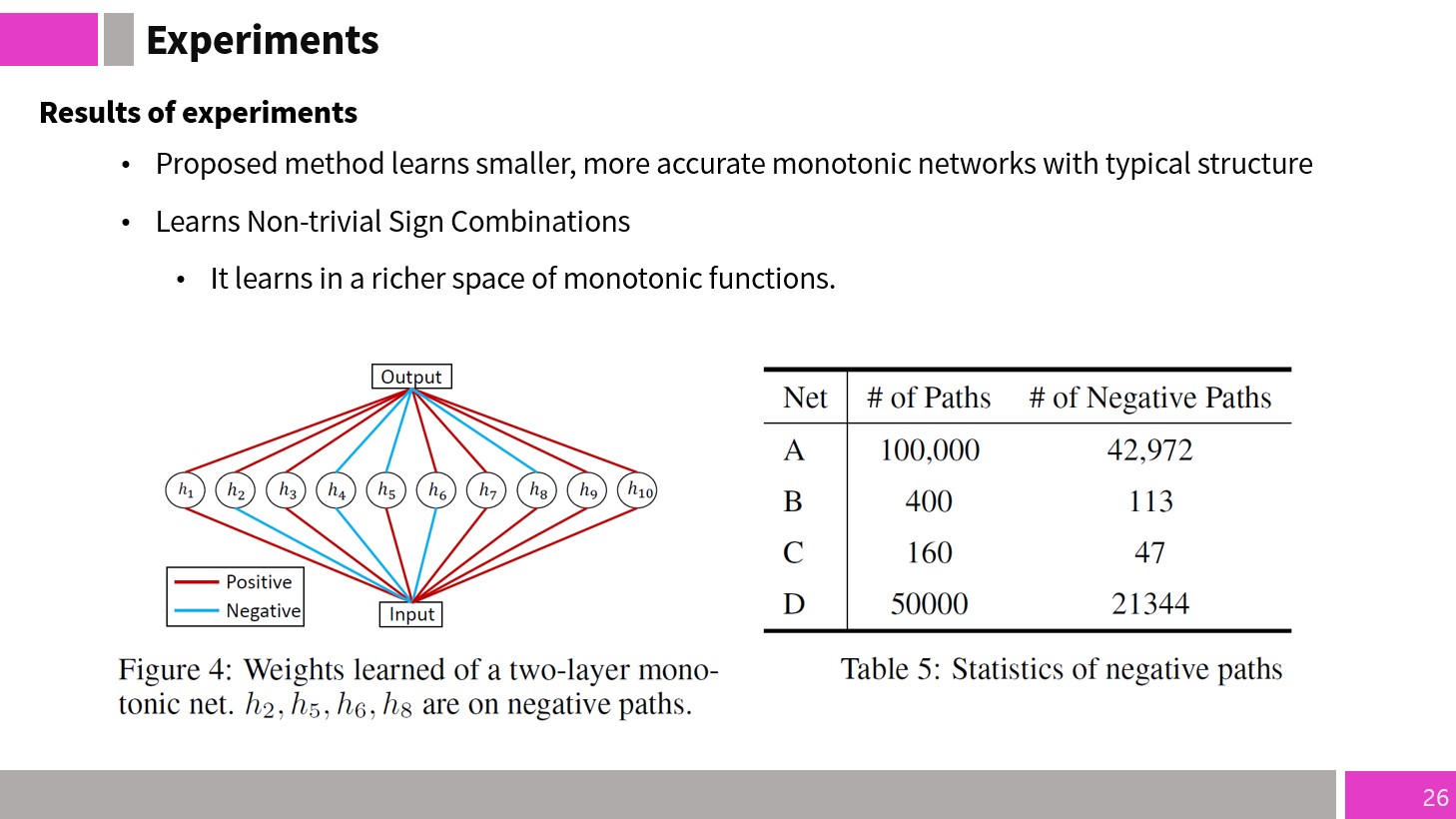
제품 정보
Certified Monotonic Neural Networks
Date | 2022-09-29 |
---|---|
Speaker | 김현호 |
- 이전글On Mixup Training: Improved Calibration and Predictive Uncertainty for deep Neural Networks 22.10.06
- 다음글THE LOTTERY TICKET HYPOTHESIS: FINDING SPARSE, TRAINABLE NEURAL NETWORK 22.06.23
Topic:
Certified Monotonic Neural Networks
Keywords:
interpretable deep learning,
Model generalization
Neural network,
Monotonicity,
Reference:
Liu, Xingchao, et al. "Certified monotonic neural networks." Advances in Neural Information Processing Systems 33 (2020)
첨부파일
-
Certifeid Monotonic Neural Network.pptx (1.5M)
47회 다운로드 | DATE : 2022-09-30 15:29:12
관련링크
저작자표시-비영리-동일조건변경허락(BY-NC-SA)
저작자와 출처 등을 표시하면 저작물의 변경, 2차적 저작물의 작성을 포함한 자유이용을 허락합니다.
단 영리적 이용은 허용되지 않고 2차적 저작물에는 원저작물에 적용된 라이선스와 동일한 라이선스를 적용해야 합니다.